OpenEarthMap Updates
OpenEarthMap-SAR: Enabling all-weather land cover mapping.
Expanding the OpenEarthMap project into the SAR domain, OpenEarthMap-SAR brings sub-meter-level synthetic aperture radar (SAR) imagery to all-weather land cover mapping. Leveraging open SAR data from Umbra and Capella Space, this dataset enables high-resolution 8-class land cover mapping, ensuring robust performance in any weather conditions. Powering innovation, OpenEarthMap-SAR will be the foundation for Track 1 of the 2025 IEEE GRSS Data Fusion Contest, driving advancements in SAR-based geospatial analysis. The dataset is now available here.
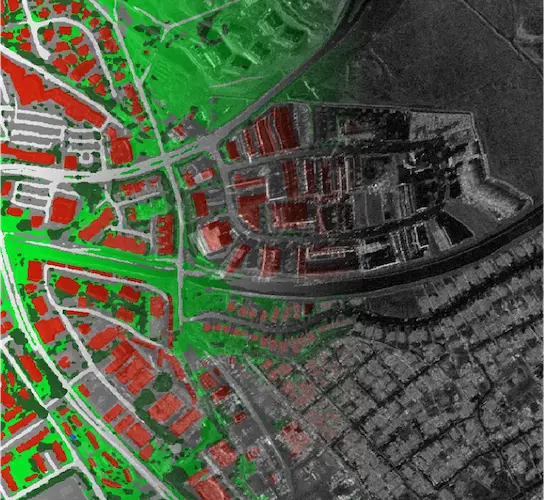
SynRS3D: Expanding OpenEarthMap into 3D.
Presented at NeurIPS 2024, SynRS3D is the largest synthetic dataset for global single-view height estimation and land cover mapping. It extends OpenEarthMap into the 3D domain, enabling large-scale semantic 3D understanding from high-resolution remote sensing imagery. To bridge the gap between synthetic and real-world data, we introduce RS3DAda, a novel multi-task unsupervised domain adaptation method that ensures effective knowledge transfer from synthetic to real-world scenarios. Both the dataset and code are now open-sourced! Get access here.
J. Song, H. Chen, W. Xuan, J. Xia, and N. Yokoya, ”SynRS3D: A synthetic dataset for global 3D semantic understanding from monocular remote sensing imagery,” Proc. NeurIPS D&B Track (spotlight), 2024.
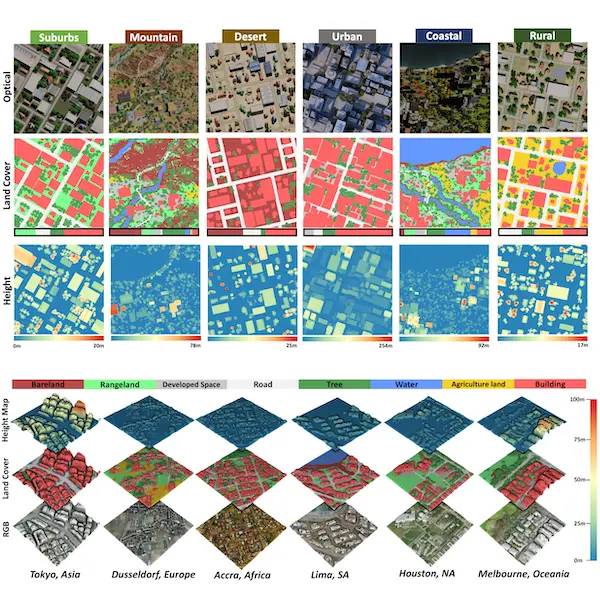
OpenEarthMap meets OpenStreetMap
Researchers have introduced a transformative approach in a study published in IEEE TGRS, using OpenEarthMap alongside OpenStreetMap data to advance land-cover change detection. Their novel object-guided Transformer (ObjFormer) seamlessly integrates object-based image analysis with advanced Transformer architecture, drastically reducing computational overhead without additional parameters. The study pioneers a semi-supervised semantic change detection task, eliminating the need for manually annotated labels and improving scalability. The code and dataset have been open-sourced and are available here.
H. Chen, C. Lan, J. Song, C. Broni-Bediako, J. Xia, and N. Yokoya, ”Land-cover change detection using paired OpenStreetMap data and optical high-resolution imagery via object-guided Transformer,” IEEE Transactions on Geoscience and Remote Sensing, 2024.
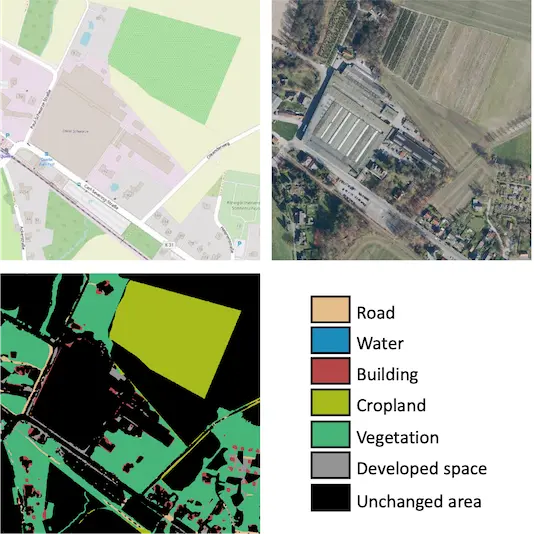
OpenEarthMap Few-Shot Challenge @ L3D-IVU CVPR 2024 Workshop
The OpenEarthMap Few-Shot Challenge, as part of the 3rd Workshop on Learning with Limited Labelled Data (L3D-IVU) at CVPR 2024, aims to advance few-shot semantic segmentation in remote sensing. It focuses on developing algorithms that require minimal labeled data, addressing key remote sensing challenges in disaster response, urban planning, and resource management. Top submissions will be presented at the CVPR 2024 Workshops and contribute to AI research for social good. More details can be found here.
C. Broni-Bediako, J. Xia, J. Song, H. Chen, M. Siam, and N. Yokoya, ”Generalized few-shot semantic segmentation in remote sensing: Challenge and benchmark,” IEEE Geoscience and Remote Sensing Letter, 2024.
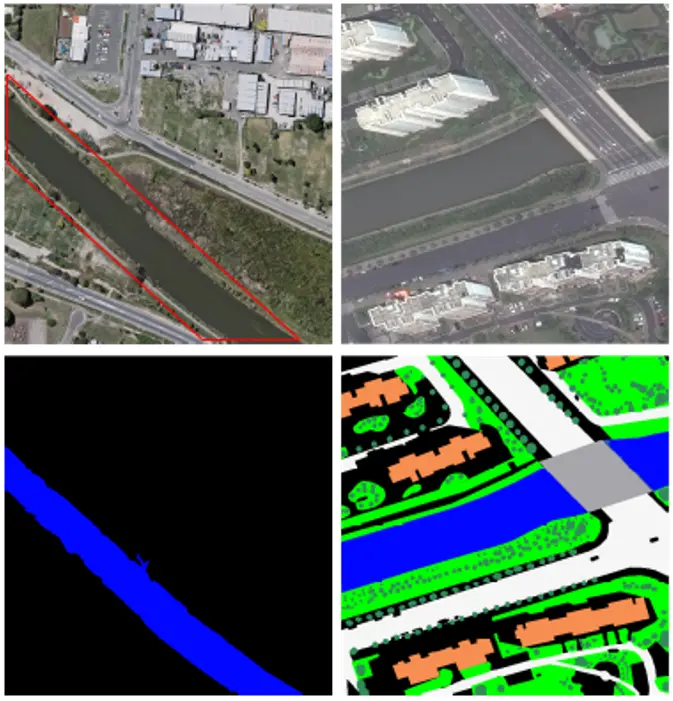
OpenEarthMap Japan: 1st national-scale submeter-level land cover map.
OpenEarthMap Japan unveils the first national-scale submeter-level land cover map, revolutionizing the way we understand Japan's diverse landscapes. This pioneering project integrates minimal additional labeled data with the extensive OpenEarthMap dataset. Highly accurate land cover classification maps across the entire country, achieving an impressive 80% overall accuracy. The map is accessible here.
N. Yokoya, J. Xia, and C. Broni-Bediako, ”Submeter-level land cover mapping of Japan,” International Journal of Applied Earth Observation and Geoinformation, vol. 127, p. 103660, 2024.
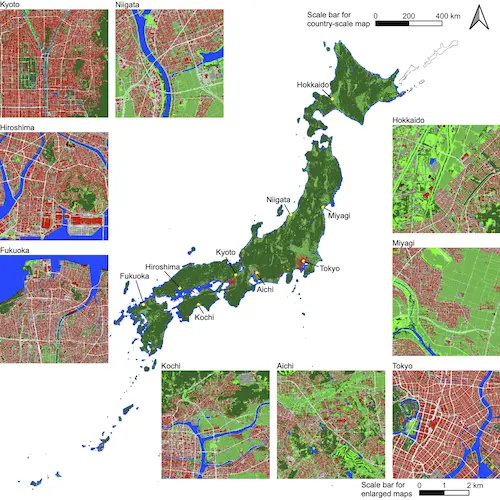
SyntheWorld: A synthetic data extending OpenEarthMap.
Reseachers developed a remote sensing image synthesis system based on procedural modeling and generative AI models. A large-scale synthetic dataset, called SyntheWorld, was constructed for land cover mapping and building change detection to improve generalization when trained together with real data. The dataset is accessible here.
J. Song, H. Chen, and N. Yokoya, ”SyntheWorld: A large-scale synthetic dataset for land cover mapping and building change detection,” Proc. WACV, 2024.
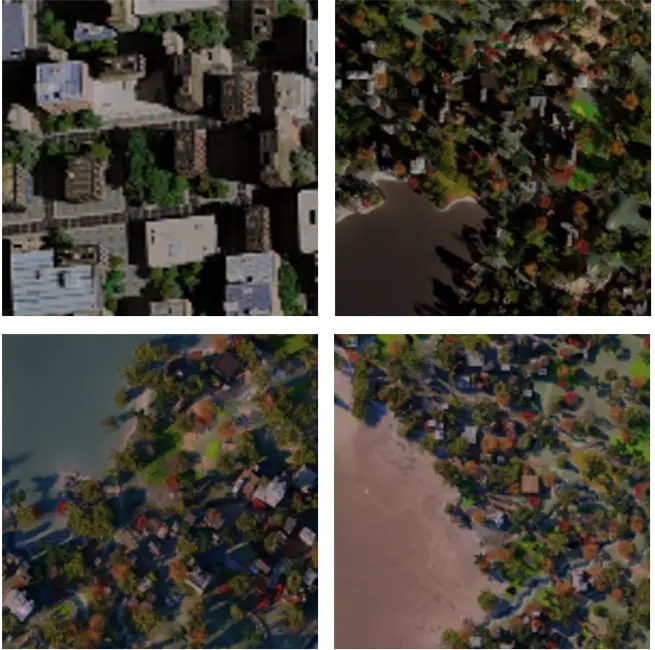